MedTechLabs Seminar: Photon-counting CT reconstruction with a deep-learning-based physics model
This thesis is a first step towards showing that deep learning model corrections are a viable way of accelerating the reconstruction process in Photon-Counting CT
Time: Thu 2021-06-17 16.00
Location: Online
Participating: Emanuel Ström, KTH
Sign up link . A confirmation will be sent to you directly together with an on-line link to the seminar.
Abstract
Photon-Counting CT is a rapidly developing alternative to conventional CT that promises higher spatial resolution, more accurate material separation and more robust reconstructions. A challenge when developing Photon-Counting CT image reconstruction methods is accounting for nonidealities in the image acquisition, such as cross-talk between detector pixels, which can generate artifacts if not corrected for. In this thesis, we accelerate image reconstruction tasks for photon-counting CT by approximating the cross-talk component of the forward operator with a deep neural network, resulting in a learned forward operator. The learned operator reduces reconstruction error by an order of magnitude at the cost of a 20% increase in computation time, compared to ignoring cross-talk altogether. Furthermore, it generalises well to both unseen data and unseen detector settings. This thesis is a first step towards showing that deep learning model corrections are a viable way of accelerating the reconstruction process in Photon-Counting CT.
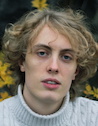
Emanuael Ström, KTH, presents his master thesis "Learning the Forward Operator in Photon-Counting Computed Tomograph"