KTH technology to map fire risk at the square meter level
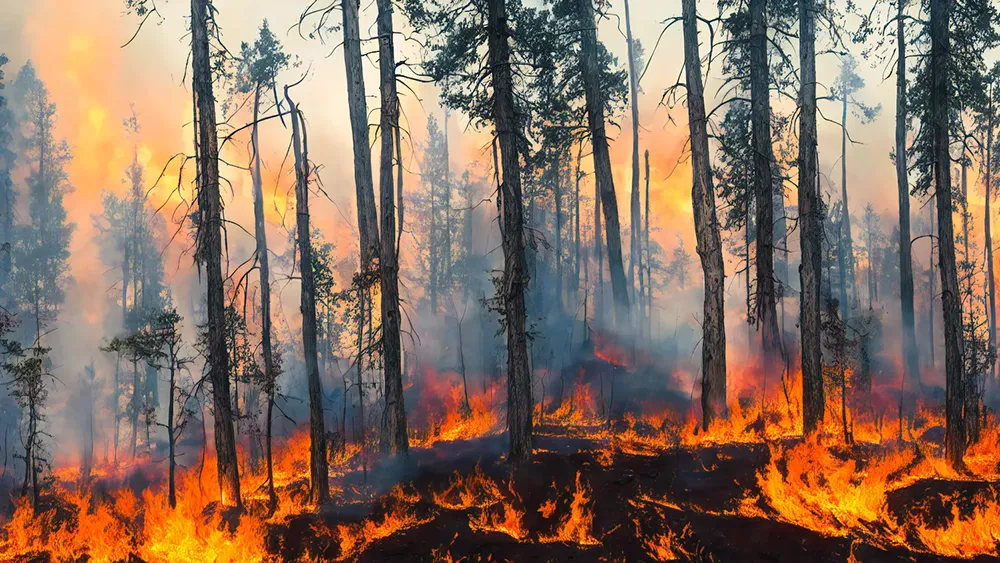
Researchers at KTH Royal Institute of Technology are working to reduce the risk of grass and forest fires in Sweden. As part of the FIRE project, they are developing a sensor platform for forestry machines that will generate high-resolution fire risk maps, providing insights at the square-meter level.
Forest fires are becoming more frequent, spreading faster and growing more intense—largely due to climate change. Led by KTH, the FIRE project aims to improve understanding of the environmental factors that contribute to fire risk. Project partners include Skogforsk and RISE.
A key part of the initiative involves producing detailed fire risk maps in areas where forestry machines operate. Doctoral student Filip Strand is developing the sensor platform that will gather the data needed to produce these maps. Designed to be mounted on forestry machines, the system will measure soil moisture levels and other risk factors in real time.
Today fire risk information is based on weather data from the Swedish Meteorological and Hydrological Institute (SMHI). The agency provides a fire danger index, which is reported in a grid dividing the country by sections measuring nearly 2.8 square kilometres each.
“The problem is that soil moisture levels vary significantly at a much smaller scale. These models assume uniform ground conditions,” he says. “But for example, a forest is usually much wetter than a clearcut area.”
By equipping forestry machines with the sensor platform, researchers will be able to collect localized data and generate detailed moisture maps. The system uses lidar technology, which employs laser beams to both map the surroundings and measure water levels in the soil. However, monitoring moisture alone is not enough to assess fire risk.
To enhance accuracy, the platform will also feature a camera with an AI-trained algorithm that detects stones—one of the main sources of sparks when metal forestry equipment comes into contact with rocky surfaces.
“Of course, avoiding rocks completely isn't possible,” Strand says. “But by classifying different types of stones, we hope to identify which ones are more likely to generate sparks.”
By combining soil moisture levels with spark risk analysis, the system can provide a highly detailed assessment of fire danger at the square-meter level.
“And because ground conditions shift throughout the day, the system can continuously update and deliver real-time fire risk data to machine operators,” he says.
While the technology is expected to be ready by 2030, widespread implementation will take longer. One of the main challenges is ensuring that key industry stakeholders invest in adopting these new safety measures.
Text: Anna Gullers