Interview with Ozan Öktem
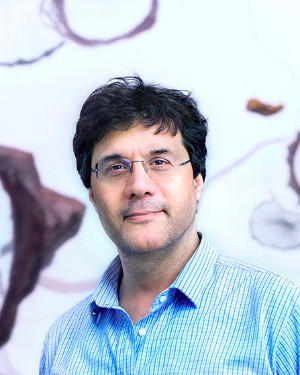
Hello Ozan, tell us a little bit about you, where do you come from, your academic studies etc.?
I did my MSc in applied mathematics at Stockholm University, where I also started my graduate studies, resulting in PhLic in 1997 and PhD in (pure) mathematics in 1999 on integral geometry and several complex variables. In parallel to graduate studies, I started in 1997 to work full-time as an applied mathematician (not a programmer) in the industry. I remained in the Swedish industry for 13 years at various R&D positions, but in 2010 I decided to try my luck in academia, so I accepted a full-time (non-faculty) position at the Centre for Industrial and Applied Mathematics (CIAM) at KTH. I became a Docent in mathematics at KTH in 2012, and from 2014 onwards, I could pursue research thanks to several grants. This work led to a guest professorial appointment in 2018 at Göttingen and a Turing fellowship at Cambridge in 2018-2019. In 2019 I was offered a position as chaired professor in Edinburgh, which I accepted. I left that position for a professorial position at Uppsala University in 2020. Finally, in 2021 I accepted the position at KTH as an associate professor. Overall, I started my scientific journey in pure mathematics, and since then, I have moved towards applied mathematics.
Taking up a new position is a challenge on its own. How has your time as an associate professor been so far, any significant changes compared to your previous position?
Bearing in mind that I have spent a long time doing research and teaching at KTH, there are not that many significant changes compared to my previous position.
What have been the most significant challenges?
Managing recruitment for ongoing projects while changing affiliation does come with some administrative challenges.
Tell us about your research; what makes it essential?
My research is about developing mathematical theory and algorithms for solving inverse problems, which means recovering a hidden model parameter from data representing noisy indirect observations. Such problems arise in various applications, like tomographic imaging. Solving an inverse problem corresponds roughly to running a simulator 'backwards', a procedure that is often ill-posed, meaning that there can be multiple solutions consistent with data and/or solutions are overly sensitive to (small) variations in data (instability). A crucial part of my research aims to handle the intrinsic instability in such ill-posed problems. The methods are based on mathematical analysis, numerical analysis, statistics, and machine learning lately.
What are the biggest challenges your research combats?
To biggest challenge is to make a scientific contribution to both mathematics while addressing a concrete problem from the scientific/industrial application. This requires one to identify high-impact challenges in applications that can be solved within a reasonable time and that require the development of new mathematical theory.
Does your work have practical applications, if so, what would those be?
My work has always been guided by the ambition to meet challenges in applications. Emphasis has been on tomographic imaging with very noisy and/or missing data and where a solution must be provided in a timely manner.
Has your work been affected by the pandemic, and if yes, how?
My clinical collaborations related to medical imaging have been adversely affected since clinicians have, for natural reasons, been given other priorities during the pandemic. Likewise, research visits and international recruitment of post-docs is more difficult due to covid-related travel restrictions.
Why did you choose your field of research in particular?
During my thesis work, I got the chance to see the interplay between pure mathematical research and applications. Next, the pure mathematical research in my PhD thesis was inspired by problems in tomographic imaging. Given my interest in applied mathematics, it was natural for me to venture into mathematical problems in bioimaging. The opportunity came in 2000 when I left a well-paid job finance for a position in a start-up. Here, I was fortunate enough to work closely with academia for an extended time, an experience that further reinforced my interest in academic research related to the mathematics of inverse problems.
What made you interested in your field?
The main driver has been the potential for working on high impact problems in sciences, and bioimaging, in particular, while contributing to mathematical research.
Is it important for you to communicate your research to the world? If yes, why and how do you do that?
It is important to communicate one's research through various channels. This is, in particular, the case for me as my work relies on using a broad range of techniques from mathematical sciences, many commonly considered as pure mathematics. However, I have unfortunately been excessively lazy in promoting my research beyond ensuring that my publications appear in GoogleScholar.
What would be your message or advice to youngsters who are interested in pursuing scientific professions?
My 13 years as an applied mathematician in the industry and my challenge-driven academic research in applied mathematics have been sobering experiences. A key element in pursuing such work is accepting that the application's problem formulation is given. Hence, unlike pure mathematical research, one has no control over the problem formulation. Next, fancy mathematical solutions are surprisingly often not competitive against simpler engineering approaches. Finally, addressing high-impact challenges often requires one to combine various methods from mathematical sciences. In particular, get prepared to continually leave your comfort zone. In retrospect, the ability to do so has proven invaluable for my applied mathematical research since many of the challenges require combining theory and methods from a broad range of mathematical disciplines. Concretely, avoid the temptation as a graduate student to only take courses directly related to your thesis subject. Allow for taking courses that broaden your horizon; mathematics is, at the end of the day, a language for communicating precise knowledge.
Text: Elina Charatsidou