Congratulations Anders Elowsson and Anders Friberg!
Best Paper Award
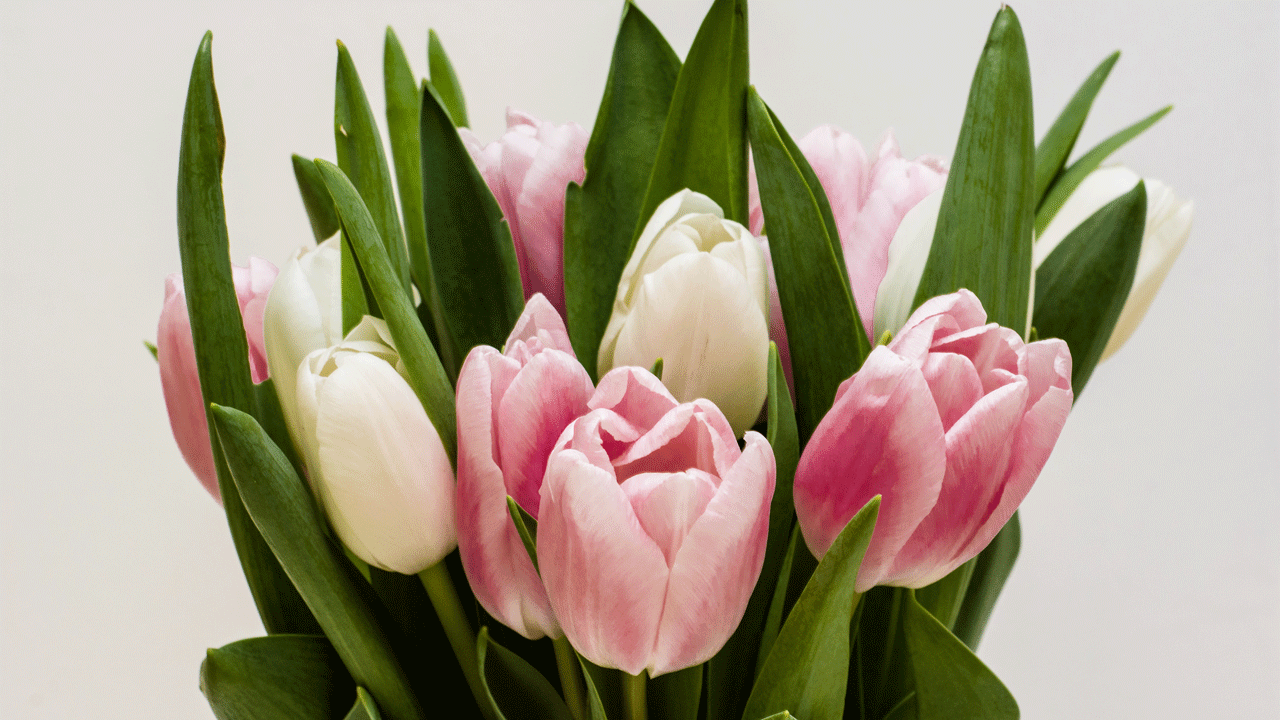
Anders Elowsson and his co-author Anders Friberg have received an ISMIR Best Paper Award for their paper "Modelling Music Modality with a Key-Class Invariant Pitch Chroma CNN". Anders Elowsson answers some questions.
Congratulations for winning the best paper award at the International Society for Music Information Retrieval (ISMIR) conference! How does it feel to have received this award?
Thank you! It feels great to get recognized in a high-impact conference and that the research methodology is appreciated.
What are you currently working with?
I have just started my employment at RITMO Centre for Interdisciplinary Studies in Rhythm, Time and Motion at the University of Oslo as a postdoctoral research fellow. Here I will continue my research concerning the analysis of music with machine learning. The objective is to further develop my music transcription systems and to apply these to gain a deeper understanding of the language of music, in collaboration with musicologist.
Tell us a little about your paper.
This paper deals with perceived modality in music, referring to if the music is in minor or major mode. In essence, we have created a convolutional neural network (CNN) that can analyse a music audio file and predict the modality a human listener will perceive. The CNN was designed to combine tones that belong to the same pitch class since these tones have a similar musical function regardless of the octave they are played at. Various key classes are also combined through max-pooling. This makes the analysis invariant with regards to key class, which is beneficial since we perceive the same modality in music regardless of if a song has been performed in for example Cm, Dm or Em. Another interesting part of the design is that we used predictions from my polyphonic pitch tracking system as input instead of the spectrum, which enabled us to better design the CNN to account for the above-mentioned musical invariances. I usually refer to this technique as “deep layered learning”.
The average rating of modality from 20 listeners for around 200 music examples was used for training. The system was able to make predictions that were closer to such average ratings than the ratings of individual listeners. In other words, you get a better estimate of the average perceived modality by asking the system than if you ask a human (at least for the type of classical film music and synthesized popular music of the dataset).