Mathematics of Data and AI
Interview with Katharina Jochemko, Kathlén Kohn Martina Scolamiero and Liam Solus, who are Assistant Professors at the newly established division "Mathematics of Data and AI" which is funded by WASP and Brummer & Partners MathDataLab.
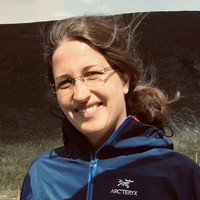
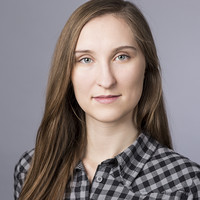
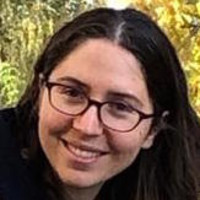
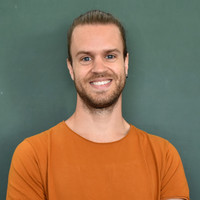
When did you start working at KTH?
Martina: I started working at KTH as a PhD student in 2013 but first visited Sweden in 2011 to participate to the thematic semester ‘Algebraic geometry with a view towards applications’ at Mittag Leffler Institute.
Katharina: I first came to KTH as a postdoctoral research fellow in 2017. In December 2019 I started my current position as an assistant professor.
Kathlén: I started working at KTH as an assistant professor in September 2019. Before that, I had been an Erasmus exchange student in the joint Master’s program on maths at KTH/SU in the fall term 2014.
Liam: I came to KTH as a postdoctoral research fellow in September of 2016, and I started as an assistant professor in September of 2019.
Where did you work before you came here to Sweden?
Martina: I pursued a PhD in Engineering at Politecnico di Torino and a PhD in Mathematics at KTH. I then was a postdoctoral researcher at EPFL in Lausanne and at the Brummer and Partners MathDataLab at KTH before becoming Assistant Professor.
Katharina: After completing my PhD at FU Berlin I held a postdoctoral position at TU Wien, Austria, before I came to KTH. In 2019 I was a Microsoft Research Fellow at the Simons Institute for the Theory of Computing, Berkeley, US.
Kathlén: I obtained my PhD from TU Berlin in July 2018. Afterwards I was a postdoctoral researcher at the Institute for Computational and Experimental Research in Mathematics (ICERM) at Brown University and at the University of Oslo.
Liam: I completed my PhD at the University of Kentucky in Lexington, Kentucky, USA in December of 2015. I was a postdoctoral research fellow at the Institute of Science and Technology Austria (IST Austria) from January 2016 until September 2016. I was also a visiting researcher at LIDS at MIT from February 2016 until May 2016.
How do you like it here in Sweden and KTH?
Martina: I truly enjoy Swedish working culture and lifestyle. I also share and appreciate values of the swedish society such as respect, equal opportunities, sustainability and the central role of children in our everyday life. This is why I think this a great place for my family.
Katharina: I very much enjoy living in Sweden. I love being outdoors, and especially hiking in the beautiful Swedish nature. It is exciting to be a part of the department of mathematics at KTH which is very international and world class in so many areas.
Kathlén: I fell in love with Stockholm when I visited the very first time in 2009 and then later again in 2014 when I met my now-husband in a Stockholm pub ;) I love the vast Swedish nature and exploring it during hikes and bike tours. The math department(s) at KTH (and also SU of course) are great, having a strong and varied research; most of all I love the international working culture.
Liam: I love living in Sweden, and in particular, in Stockholm. Stockholm is one of my favorite cities in the world. It has a special way of blending nature with city life that you cannot find anywhere else. KTH has a fantastic mathematics department, and its strong combinatorics group is what drew me here in the first place. Now, thanks to the joint efforts of KTH and WASP, the institute for mathematics at KTH also offers one of the best opportunities for young researchers interested in the mathematical foundations of data and artificial intelligence. It has been so much fun these past few months being a member of our quickly growing Mathematics for Data and AI division. I’ve never been more excited for the future!
How do you handle the new pandemic situation in combination with the work?
All: The new division for Mathematics of Data and AI at KTH is rapidly growing and already in excess of twenty members after only its first year. Most of these new members (including the four of us) are quite young and excited about starting new collaborations. One consequence of this is that members of our group seemed to always be around the department, chatting in hallways or the lunchroom and writing on available chalkboards. This sort of interaction was, of course, limited by the pandemic once we had to start practicing more extreme forms of social distancing. However, many of the members still found ways to meet and chat while staying safe and following department regulations. Among other things, this included attending our seminar Applied CATS (short for Applied Combinatorics, Algebra, Topology and Statistics) via a hybrid setting: a limited number of people could attend in-person and any overflow audience was invited to attend online via Zoom. For now, the seminar continues solely online, but we are looking forward to returning to the hybrid set-up once the pandemic conditions allow for it.
Tell us about your new research group Mathematics of Data and AI section.
All: The division "Mathematics of Data and AI" is formed by the four of us, together with all PhD students and postdocs at the math department that are funded by WASP or the Brummers & Partners MathDataLab. We are one of the 5 divisions at the Department of Mathematics. Our head is Johan Håstad, and we started our division in December 2019. Now our division has already more than 20 members! Our division is a highly international, gender-diverse group, which, by nature, is also mathematically diverse and targeted at interdisciplinary collaboration. Each of us four new assistant professors has a different background in theoretical mathematics: discrete geometry (Katharina), algebraic geometry (Kathlen), combinatorics (Liam), and topology (Martina). Similarly, our division head, Johan Håstad is an expert in complexity theory and cryptography. Even more, each of us has a goal of connecting our respective field in mathematics with the new and exciting developments in data science and artificial intelligence. The result is a lot of exciting interdisciplinary interactions, which are show-cased in our weekly Applied CATS seminar and driven by our collaborations and the collaborations of our new postdocs and PhD students.
Please tell us about your research and how it connects to WASP.
Kathlén: The WASP program is a world-wide unique investment in the mathematical foundations of data and AI that recognizes the importance of many different areas of math in applications. Something that is so unusual. For a researcher like me - working on both “pure math” (namely algebraic geometry) and applications - it can be hard to find my place in a math department: sometimes I am considered too applied by certain groups or too pure by others. The WASP program and our division on Math of Data & AI provides this place naturally.
For me it is very important to recognise that pure math can play a role to help build a common ground and common language between different research communities. I am actually dreaming of that one day we can overcome the historical borders between “pure” and “applied” math. And I believe that WASP can help as it spans the whole spectrum, from all sorts of math via engineering sciences to industry. And WASP organizes many joint events to facilitate communication across fields. This creates unique opportunities for interdisciplinary collaborations which is so important to really make a difference.
Martina : My research is in Topological Data Analysis (TDA) and I fit into the larger reality of the TDA group at the Mathematics department of KTH. In TDA we use topology to study spaces arising from data. We extract geometrical features that can then be used for statistical analysis and machine learning. Growing evidence from different fields such as neuroscience, and material science or image recognition and sensor coverage analysis shows that such geometrical features are actually important to understand the data.
The WASP environment offers me the unique opportunity to meet leading experts in A.I. which allows me to both improve my mathematical methods and find test-beds to try them. Furthermore the WASP graduate school provides a great education platform for our PhD students. I am contributing to this graduate school by offering a TDA course together with W. Chacholski and F.Pokorny. This course is based on novel mathematics developed by us.
Liam: My research is in combinatorics (or discrete mathematics) and its applications. Most of the problems I focus on center around how we can use combinatorial objects, such as networks, permutations, and high-dimensional generalization of polygons (called polytopes), to encode, describe, represent, or even learn important features of a probability distribution. When working with data, we often assume that the samples we have are drawn from some unknown probability distribution. A major goal of my work is to find ways to represent important features of this unknown distribution with a combinatorial object. Oftentimes, we end up using one combinatorial object, like a polytope, to learn a different combinatorial object, such as a network, that best represents what our data is telling us about the unknown distribution. One key example of this is the problem of causal discovery , in which we aim to learn a network that best encodes the cause-effect relations among a family of random variables (or events). Currently, I am interested in identifying new ways that combinatorics, and its connections with algebra and geometry can help us address this problem. Causality is one of the cornerstone fields of research in modern machine learning and AI, and hence WASP has created a lot of opportunities for me to connect with industry researchers interested in the subject. This includes my new industrial PhD student, funded by WASP and our industry collaborator Combient MIX. Aside from working to create new mathematics for data and AI, I am also interested in what directions new results in AI suggest for mathematics. So I also spend a good deal of time thinking about new, theoretical, combinatorial problems that are motivated by recent results in causality and AI.
Katharina: My research interests lie at the intersection of discrete mathematics and geometry. In particular, I study combinatorial aspects of polyhedra which are geometric objects given by finitely many linear constraints. Linear separation of data is a fundamental concept in machine learning and thus many AI systems possess underlying polyhedral structures. The WASP environment creates unique opportunities to interact with leading experts in AI from academia as well as industry. This fosters inspiration for my theoretical research as well as possible application areas and facilitates new collaborations.
What is your contribution in artificial intelligence and how do you think it can contribute to society?
Kathlen: My main area of expertise is algebraic geometry and its applications. Within AI, my research focuses on 3 areas: computer vision, machine learning, and statistics. In computer vision, I am mainly interested in understanding how to reconstruct 3D scenes from 2D images. This appears in many modern applications, such as self-driving cars, the movie industry or medicine. I use my algebraic geometry tools to explain why certain reconstruction methods work and why others don’t; and also how well different methods work when it comes to running time and numerical stability. So my research can guide those that implement solvers for reconstruction tasks to estimate which strategies might be practically feasible.
In machine learning, my research focuses on explaining behaviors of neural networks that are otherwise only observed experimentally. Every machine learning problem has an underlying intrinsic geometric structure which I believe governs WHY certain behaviors are expected. If we would have a better understanding of these intrinsic reasons, then we could make deterministic choices when setting up neural networks instead of just tweaking parameters until the results look good. And, maybe even more importantly, we could interpret the results of neural networks in a better way, which is essential for explainable AI.
Finally, in statistics, I am mainly working on maximum likelihood estimation. This is a method that is very closely related to machine learning with neural networks, as both approaches try to find a best distribution explaining some training data.
Katharina: Many aspects of our today’s life are shaped by AI based algorithms, such as search platforms, translation websites, dating apps or spam filters. While technological advancements are made on a high speed our fundamental understanding of how and why these systems work is still in its infancy. With my research I hope to contribute to a better mathematical understanding of such systems.
Liam: My main contributions to the field of artificial intelligence are within the subfield of causality (as mentioned in my response to the previous question). In causality we are interested in learning the cause-effect relations among a system of random variables (or events) based on the available data. Most of my work in this area has focused on how we can use modern combinatorics, and its connections with algebra and geometry to discover the causal structure (direct cause-effect relations) within a complex system. The networks we learn using various combinatorial methods can then be used by applied scientists to infer the strength of the causal effect of one variable on another and make predictions. This has applications in a wide variety of areas within AI and data science as well as in biology, sociology, economics and many more fields of study.
Martina: A central idea at the core of my research is to be able to identify TDA invariants which smoothly fit in an A.I pipeline. For example one of the invariants I have introduced during my PhD, the ‘stable rank’, has a strong potential to be used in supervised learning. Together with other members of the TDA group we are working on understanding how to improve its discriminatory power in classification tasks by learning appropriate ways to encode noise in the system. In order to have robust and replicable findings I have also worked on defining a machine learning algorithm based on a topological map of the data. This research led to the publication in Nature Molecular Psychiatry: ‘Topology predicts long term functional outcome in early psychosis’ in collaboration with F. Margot, K.Hess, K.Do et.al.. Here the topological map of the data allowed us to identify a subgroup of Early Psychosis patients with a unique clinical profile which leads to good outcome after a three years clinical program. In this type of application topology was essential in finding a non linear combination of patients features which outline the clinical profile. More generally this type of tool can be used for a better understanding of medical and biological data, with the hope of contributing to public health.
What are you doing for the moment?
Katharina: My first PhD student and postdoc, both funded by WASP, started their positions at KTH in October. Together with my postdoc I am currently investigating questions arising in geometric tomography where the task is to recover an object from geometric data such as X-rays or brightness function evaluations. My PhD student will be working with me on discrete-geometric model complexity measures that are relevant in statistical learning theory and computational geometry.
Kathlén: There are always lots of exciting things going on, that is one of the great benefits of the job. For instance, Liam and I teach a PhD course together this spring: “Combinatorial and Algebraic Statistics” which will be a lot of fun. Currently I work on 5 research projects, with my PhD students and postdocs and many brilliant collaborators from all over the world, in a variety of topics (e.g. rolling shutter cameras in computer vision, linear convolutional networks in machine learning, the Burer-Monteiro method in optimization). Together with Joakim Andén, associate professor at the statistics division, I will also hire another PhD student this year. He or she will be the 4th PhD student I (co)-supervise :)
Liam: In addition to the PhD course we will be teaching this Fall, I’m excited about a number of ongoing research projects. For instance, my new industrial PhD student funded by WASP and our industry collaborator Combient MIX started at KTH this January. His project will be focused on how we can learn causal relations and use them to make predictions within social media and mass media. On the more theoretical side, my other PhD student funded through WASP, who started just this Fall, will be working with me to explore how certain combinatorial generating functions can encode the complexity of models useful in probabilistic inference. I also have two master students this semester who I am really excited about: one will be working on a project taking a game-theoretic approach to targeted interventions in context-specific causal systems, and the other will be developing some new causal discovery algorithms for learning context-specific causal structure from data. Both of these two projects are linked to some exciting new work on modeling causal relations in context-specific settings that I recently finished with my collaborator Eliana Duarte (Otto-von-Guericke Universitat, Magdeburg, Germany).
Martina : During these days I am returning to work from parental leave. I am so grateful for having such an exciting work to go back to, that values my contribution and offers so many opportunities. One of these is being part of the dBRAIN project in KTH Digital Futures. dBRAIN is an interdisciplinary initiative to better understand neurodegenerative diseases such as Parkinson’s disease and Alzheimer’s disease. Together with W. Chacholski we will be working with experts from computational neuroscience and biomedical engineering from SciLifeLabs and KI. We will use topology to investigate structural and functional properties of brain networks and how they are affected by neurodegenerative disorders. I am also excited about an ongoing project with Jens Agerberg, WASP funded PhD student. Jens is investigating kernel methods based on the ‘stable rank’, their discriminatory power and statistical properties. These improved methods will allow us to better tackle our data analysis .